Datorios has launched new observability capabilities for Apache Flink, enhancing the monitoring and management of responsible Agentic AI, ensuring transparency and reliability in AI-driven applications.
Duncan is an experienced editor with over 20 years of work in journalism; he received numerous awards.
Having started his journalism at Arabian Computer News in Dubai he has gone on to edit many technology and digital marketing titles including Computer Business Review, TechWeekEurope, Figaro Digital, Digit and Marketing Gazette.
Datorios, the data observability and data quality technology for real-time business operations, has released new observability innovations for the open source Apache Flink stream processing system.
Unveiled at the formal Flink Forward 2024 conference in Berlin Germany, the new solution from Datorios provides businesses with the necessary visibility to ensure Flink driven real-time AI applications deliver sound, logical business automation in real-time all the time.
Datorios links information about real-time operation of a system and its data with the code and infrastructure, making it much easier to identify issues with functioning AI solutions.
It’s necessary to differentiate agentic AI from the previous levels of AI applications. Thus, while agentic AI goes even further than creating answers to questions, it involves AI in constructing and pursuing plans to achieve intended objectives.
It actually makes decisions and then controls operations to be in harmony with these decisions. Gartner stated that the bot-for-job, agentic AI will increase its capability to make the day-to-day work decisions independently and said that by 2028, 15 % of those decisions will be made by AI.
Real-time AI needs a powerful tool like Apache Flink observability
Apache Flink is one of the most important components of the real-time agentic AI architecture. It is also employed to merge and coalesce data from an entire enterprise and present the data in a form that is processed by AI agents to take an action.
Since businesses rely more and more on artificially intelligent systems, they need to be able to watch what goes on inside of Flink programs in order to ascertain what decisions an agentic AI makes.
Ronen Korman, CEO and co-founder of Datorios, said: “No business today runs without cybersecurity, and under the same rationale, no business should allow AI to run operations without observability.”
Agentic AI has a lot of positive potential for business, but you have to know how the AI systems are automating your business. Datorios provides you the visibility for the required operational quality that no business can afford to ignore.”
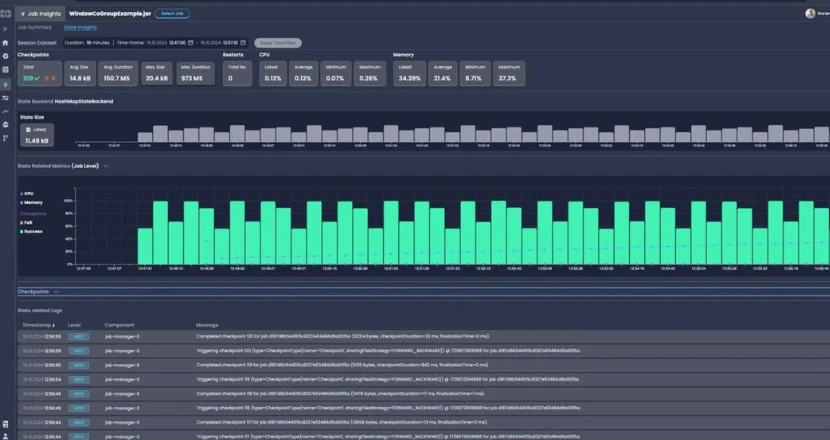
Discovery of new correlated traces and data lineage analytics for Apache Flink
Due to real-time data that enters and exits AI models like LLMs, the data is processed by Apache Flink programs. Periodically, it is required to monitor how these Flink programs are performing —in the wake of a system failure, a customer complaint or a regulatory audit request.
From Datorios the same observation capabilities are now offered in interactive dashboards suitable for real-time stream data processing in Flink.
This makes it possible for businesses to identify the source of a problem, and address it in the shortest time possible.
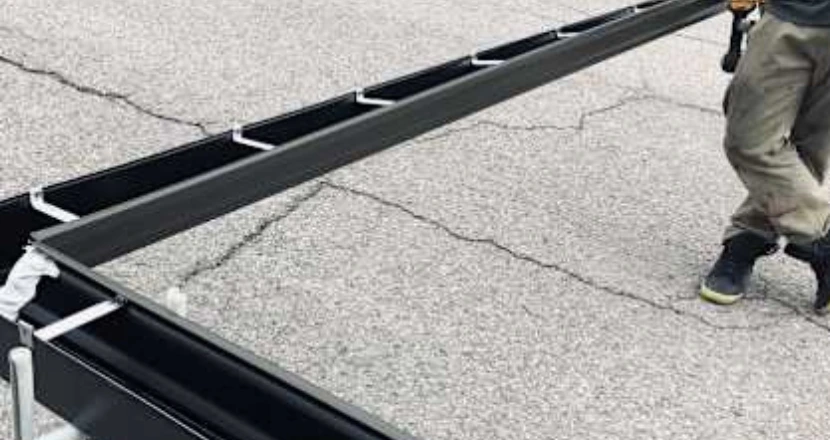
Correlated traces – the root of the AI system failure can be nearly always found in the fact that something is wrong with the data fed to the AI system, the Flink code that uses the data, or the infrastructure that Flink runs on.
Datorios ensures that data traces gathered from data, code, and infrastructure are related in one interface, which enables Flink teams to identify and solve problems efficiently.
That is why with Datorios, operational complexities are explained and Flink users are able to effectively keep the operational continuity that they require to thrive.
Through lineage, the Flink teams get to know how data flows within Flink jobs, and they can easily determine when and why data may have changed, and who in the team is most likely to address the code or data source that caused the problem in the shortest time possible. Lineage analytics capabilities include:
Lineage graphical representation – A live Job Graphs to the individual operator level within a task.
Data flow detail – Use Job Graphs for navigation to more specific views of a downstream data flow and of transformations.
Data validation – Carry out investigations above your data and logics that are involved in handling breaks of data.
Low performance overhead – Linear Analyzer runs as a separate process from you Flink jobs, the result being that you can achieve the necessary visibility without negative influence on performance of your real-time system.